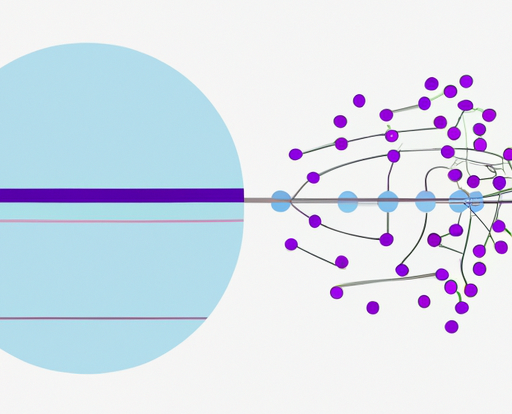
Imagine a world where machines can generate realistic and high-quality content, from text to images to music, without any human intervention. Sounds amazing, right? However, as with any technological advancement, there are always drawbacks to consider. In this article, we will explore the disadvantages of generative AI, shedding light on the potential pitfalls and challenges that come with this revolutionary technology. From ethical concerns to the risk of misinformation, join us as we delve into the complexities of generative AI and uncover the downsides that accompany its many benefits.
Bias and Discrimination
Generative AI, while a breakthrough in technology, is not without its disadvantages. One major concern is the pre-existing bias that can be found within the training data used to train these AI models. Training data often reflects the biases present in society, as it is derived from real-world examples. As a result, generative AI models may inadvertently learn and replicate these biases, leading to discriminatory outcomes. For example, if the training data contains predominantly male faces, the AI model may generate predominantly male faces and exclude the representation of other genders. This can perpetuate existing biases and contribute to the marginalization of certain groups.
Furthermore, generative AI has the potential to amplify biases already present in society. By learning from biased data, AI models can inadvertently reinforce and perpetuate harmful stereotypes. For instance, if the training data shows a disproportionate representation of certain ethnicities in certain occupations, the AI model may generate outputs that further reinforce such stereotypes. This amplification of biases can have far-reaching consequences in perpetuating systemic discrimination and limiting opportunities for marginalized communities.
Overdependence and Loss of Human Skills
Another significant disadvantage of generative AI is the potential overdependence on AI-generated outputs, leading to reduced human input. As AI models become more sophisticated and capable of generating content, there is a risk that human involvement and decision-making may diminish. This overreliance on AI-generated outcomes can undermine the valuable skills and expertise that humans bring to the table. It is crucial to strike a balance between utilizing generative AI and maintaining human involvement to ensure a comprehensive and nuanced approach.
Moreover, the introduction of generative AI can lead to a diminished level of creativity and innovation. AI models are trained on existing data and patterns, which limits their ability to think outside the box and generate truly novel and groundbreaking ideas. Human creativity, on the other hand, thrives on imagination, intuition, and the ability to connect disparate ideas. The reliance on generative AI could stifle these crucial human skills, hindering the potential for innovative breakthroughs and novel solutions to complex problems.
Additionally, the widespread implementation of generative AI has the potential to lead to significant unemployment. As AI models become more proficient in generating content, there is a concern that certain industries and job roles may become obsolete. For example, if AI can autonomously create art, write articles, or compose music, it may eliminate the need for human artists and writers. This displacement of workers can have severe socioeconomic ramifications and calls for thoughtful consideration of the balance between automation and human involvement.
Ethical Challenges
The ethical implications of generative AI cannot be overlooked. One prominent ethical challenge is the lack of accountability and responsibility in AI-generated outputs. When an AI model generates content, it can be difficult to attribute ownership or assign responsibility for any potentially harmful or erroneous outputs. This lack of accountability raises important questions about who should be held responsible for the consequences of AI-generated content.
Furthermore, the ownership and copyright of AI-generated works pose ethical dilemmas. As AI models increasingly create content, such as music, art, and writing, questions arise regarding the rightful ownership of these creations. Should the creators be credited, or should the AI algorithms be recognized as the creators themselves? This raises complex ethical issues surrounding intellectual property rights and the attribution of creative works, warranting careful examination and consideration.
The decision-making capabilities of generative AI also give rise to ethical dilemmas. AI algorithms make decisions based on patterns and data, but these decisions may not always align with ethical or moral standards. For instance, an AI-driven decision-making process may inadvertently prioritize certain demographics or perpetuate harmful biases. The ethical implications of these decisions underscore the importance of robust ethical frameworks and conscious decision-making to mitigate any potential harm.
Data Privacy and Security Risks
Generative AI relies heavily on vast amounts of training data, raising concerns about data privacy and security risks. Large datasets used to train AI models can be susceptible to breaches and unauthorized access, potentially compromising personal and sensitive information. The unauthorized use or exposure of such data can have severe consequences, including identity theft, fraud, and other privacy infringements. Adequate security measures must be in place to protect these valuable datasets from unauthorized access.
The issue of data bias is another concern in generative AI. AI models trained on biased data can perpetuate and amplify biases in the generated outputs. For example, if training data contains historical societal biases or discriminatory patterns, the AI model may produce biased results that reflect those patterns. This poses a threat to fairness and equality, further reinforcing existing power imbalances and discrimination. Safeguards and measures should be implemented to address and mitigate data bias, ensuring equal representation and fair outcomes.
Additionally, generative AI can make systems more vulnerable to cyber attacks. As AI models become more prevalent and interconnected, they create potential entry points for malicious actors to exploit. Cybercriminals may attempt to manipulate AI-generated outputs to spread disinformation, engage in identity theft, or disrupt critical infrastructures. The security of AI systems is of utmost importance to prevent unauthorized access and protect against potentially devastating cyber attacks.
Robustness Issues
The robustness of generative AI systems is a significant concern and can impact their reliability and trustworthiness. One common issue is their sensitivity to input variations. Small changes in input can lead to significant variations or inconsistencies in the generated outputs. This lack of stability can hinder the effectiveness and reliability of AI models, making them less dependable in real-world applications. Ensuring robustness and stability is paramount to instill confidence in the outputs generated by AI systems.
Another challenge is the susceptibility to adversarial attacks. Adversarial attacks exploit vulnerabilities in AI models to deceive or mislead them. By making slight modifications or introducing imperceptible changes to the input, attackers can manipulate the generated outputs. Adversarial attacks can have serious implications, particularly in critical domains like autonomous vehicles or cybersecurity systems. Developing robust defenses against adversarial attacks is essential to maintain the integrity and reliability of generative AI systems.
Furthermore, the lack of explainability and trustworthiness is a significant drawback of generative AI. The inner workings of AI models can often be complex and difficult to understand. As a result, it can be challenging to explain or justify the decisions made by these systems. This lack of transparency hampers the trust that users, regulators, and the general public have in AI-generated outputs. Establishing interpretability and explainability mechanisms is crucial to foster trust and confidence in the outputs of generative AI systems.
Unreliable and Inaccurate Outcomes
Generative AI can suffer from unreliable and inaccurate outcomes, posing a significant challenge in its widespread adoption. These systems have the potential to generate false or misleading information, leading to the dissemination of inaccurate content. For instance, AI models used in generating news articles may inadvertently generate fake news or biased information, causing harm to individuals and society at large. Ensuring the accuracy and reliability of the outputs generated by AI systems is vital to maintain public trust and prevent misinformation.
Moreover, generative AI systems may struggle to handle complex situations that require nuanced judgment and contextual understanding. While AI models can excel in certain tasks, they often lack the ability to fully comprehend complex scenarios or understand subtle nuances. This limitation can result in incomplete or inappropriate responses in situations that demand a comprehensive understanding of the context. Utilizing generative AI within appropriate boundaries and ensuring human oversight is essential to mitigate the risks associated with inaccurate or inadequate responses.
Furthermore, the behavior of generative AI systems can be unpredictable. Due to their reliance on complex algorithms and training data, AI models may produce unexpected or unintended outputs. This unpredictability can lead to unintended consequences or pose risks in critical applications. Understanding and managing the potential for unpredictable behavior is vital to avoid any adverse outcomes and ensure the safe and responsible use of generative AI.
Resource Intensive and Environmental Impacts
The adoption of generative AI comes with resource-intensive requirements, posing challenges in terms of computational resources and environmental impacts. Training AI models often demands extensive computational power and infrastructure. High-performance computing resources are necessary to train complex models effectively. The accessibility and affordability of such resources can pose barriers to entry for individuals or organizations with limited access to these resources.
Moreover, the energy consumption associated with training and running AI models can have a significant carbon footprint. Large-scale AI models can require substantial amounts of electricity, contributing to greenhouse gas emissions and environmental degradation. Considering the environmental impact of AI systems and exploring energy-efficient alternatives are crucial steps towards ensuring sustainability and minimizing their carbon footprint.
Additionally, the rapid development and adoption of generative AI technologies contribute to the generation of electronic waste (e-waste). As AI hardware and infrastructure become outdated or replaced, the disposal of these electronic components can strain waste management systems and pose environmental hazards. Responsible disposal and recycling practices are essential to mitigate the environmental impact and reduce the accumulation of e-waste.
Limited Control over Model Outputs
One of the challenges in leveraging generative AI is the limited control over model outputs, which can have implications in various domains. Steering the outputs of AI models to align with specific requirements can be a complex task. Despite guidelines or instructions given during the training process, AI models may not always produce desired or expected outcomes. This lack of control can limit the practical applications of generative AI, especially in areas where precision and customization are essential.
Moreover, generative AI systems often lack subjectivity and contextual understanding. While they can process vast amounts of data and learn patterns, they may struggle to capture the nuances of subjective experiences or interpret contextual cues accurately. This limitation can hinder their ability to generate outputs that are in line with human preferences or tailored to specific contexts. Human judgment and intervention may still be necessary to ensure the suitability and relevance of generative AI outputs.
Additionally, the potential for malign use of generative AI is a significant concern. AI-generated deepfakes, for example, can be exploited to spread misinformation, manipulate public opinion, or engage in illicit activities. The ability to create highly realistic and convincing AI-generated content raises ethical concerns and necessitates the development of robust safeguards to prevent misuse. Responsible deployment and stringent guidelines are crucial to mitigate the potential harm arising from the malicious use of generative AI.
Legal and Regulatory Challenges
The rapid advancement of generative AI technology has outpaced the development of comprehensive legislation and regulatory frameworks, leading to legal and regulatory challenges. The absence of clear and well-defined regulations surrounding generative AI can hinder its responsible development and use. Questions regarding liability, accountability, and legal frameworks for AI-generated content remain largely unanswered. The legal landscape must adapt to address the intricacies and complexities of generative AI to ensure fair practices and protect the rights and interests of both creators and users.
Moreover, the ownership of AI-generated content raises intellectual property rights concerns. Determining ownership and attributing creative works to AI systems pose unique challenges that current legal frameworks may not adequately address. Balancing the rights of AI algorithms and human creators while ensuring fair compensation and recognition remains a complex and evolving legal area.
The issue of liability and accountability is also a significant consideration. In instances where AI systems generate content that causes harm or damage, determining who should be held responsible can be challenging. For instance, if an autonomous vehicle powered by generative AI causes an accident, should the liability fall on the AI system manufacturer, the vehicle owner, or the human user? Clear guidelines and legal frameworks are necessary to allocate liability appropriately and ensure accountability in cases involving generative AI.
Adoption and Implementation Challenges
The adoption and implementation of generative AI technologies face their own set of challenges. One key stumbling block is the cost and accessibility barriers associated with these advanced technologies. Developing and deploying generative AI systems can require significant financial investments, making them inaccessible to organizations or individuals with limited resources. The affordability and availability of generative AI technologies must be addressed to ensure equitable access and adoption.
Resistance to change is another challenge that arises when introducing generative AI. The integration of AI technologies may disrupt established workflows and traditional practices, leading to resistance from individuals or organizations who may perceive the change as threatening. Overcoming this resistance requires effective communication, transparency, and addressing concerns to demonstrate the benefits and value of generative AI in various domains.
Furthermore, successfully utilizing generative AI technologies requires expertise and training. The complexity of AI algorithms and models demands specialized knowledge and skills to develop, deploy, and maintain these systems effectively. Access to qualified experts and comprehensive training programs is imperative to ensure the successful adoption and implementation of generative AI while mitigating any associated risks or challenges.
In conclusion, while generative AI holds immense promise and potential, it is essential to be aware of its disadvantages and overcome the challenges it presents. Bias and discrimination in training data, overdependence on AI-generated outputs, ethical implications, data privacy and security risks, robustness issues, unreliable outcomes, resource-intensive requirements, limited control over model outputs, legal and regulatory challenges, and adoption difficulties are concerns that must be addressed. By acknowledging and proactively addressing these challenges, we can harness the power of generative AI for the betterment of society, ensuring responsible and ethical use while maximizing its benefits.